Customer Care Agent – a Robot?
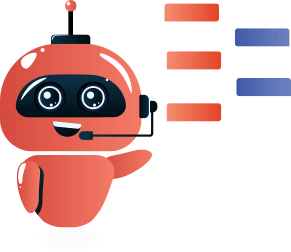
Talking is always the chosen source from the 18th century to avoid long conversation complexities. As chatbots are gaining over edge in the tech industry, talking bots are made into vogue. After gossip calls and motherly talks, customer care is the most frequent callers today. Just imagine, robots handling this department! Throw your curveballs, it’s the smart bot.
In defining the target market, you will identify key elements such as geographic location, industry, customer characteristics, your target market’s needs and how these needs are being met currently. To train such tricky bot is all new analysis to be done.
In defining the target market, you will identify key elements such as geographic location, industry, customer characteristics, your target market’s needs and how these needs are being met currently. To train such tricky bot is all new analysis to be done.
Before the technology Humans
The representatives of a major Energy firm used to get over
20,000 calls a day inviting handoffs and a lot of patience. They were stretched to thin picking up and solving a major error from day to night apart from handling other minor problems
of the day.
IndustryEnergy firm which develops Solar panels with 24 types of slot.
Major Call ReasonGetting an error pattern among the 24 slots of Solar panel.
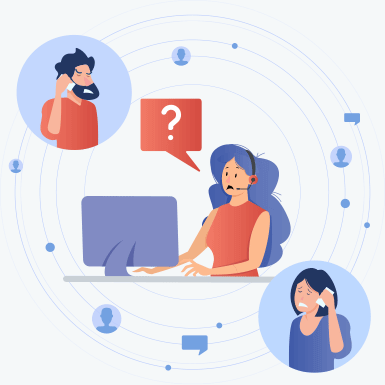
1.
Industry Aim To minimize the maximum proportion of calls received in turn reducing the pressure level on customer care representatives. Handling 20 % of the calls, can reduce up to 64% of weightage.
2.
Custom TrainingTo train the bot to gauge up with one long form of 13 pages to solve the major error faced with over 12-15 different scenarios.
3.
Boundaries Form digression, in the cases if customers do not understand the Energy jargon. Handling the training in scope of the form and its components with a repertoire of data types.
4.
The Resources Watson Assistant has been a great NLP engine with intent mapping and appropriate entities binding.
Challenges






Bot Training
My first question during the initial phase of training was “Can my bot bypass the lengthy 13 pages of action?” I had to design to function just like an understanding human. (Socially impossible :D) Providing a full fledge support rep was the aim. Understanding the different scenarios and identifying the complexities made the process easier. The first main step was to analyze the actual customer care scene.
So, these were the questions I needed an answer from:
So, these were the questions I needed an answer from:
What kind of doubts do the customer have?
Which step or scenario was the most cumbersome?
How they explained the core details of the error?
Any delayed actions?
What is the maximum wait time support?
Which are the keywords used by the customer?
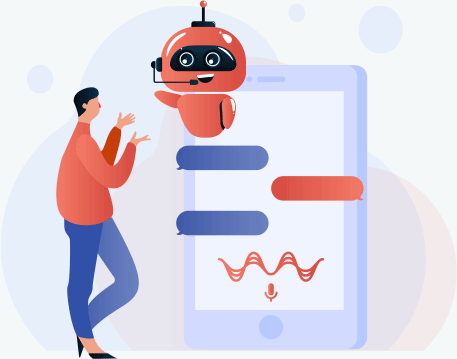
Handling the Training of Complexities
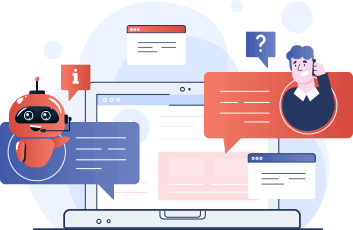
Reaching to the maximum extent of prototyping the call
conversation was the end goal. I started with jotting down the number of entities and intent requirements and the mapping criteria of the same.
Bifurcating the types of complexities which can be handled by entities and the ones which can be handled by code turns the process at ease.
The next task was to train:
Bifurcating the types of complexities which can be handled by entities and the ones which can be handled by code turns the process at ease.
The next task was to train:
The gaps and halts in the conversation: Handled by digression and introducing wait time.
Misinterpretation of words was handled by implementing a famous proverb in Hindi translated as “Let us see which way the wind blows”. I tried to interpret the major keywords and their understandings by a voice agent. Training the reverse flow narrowed the slit and broadened the word limits.
Many types of customers could dial the support team: the first-time visitors, the used to customers, the pro users. Knowing the type and responding was the approach by which I trained.
Continuous recording and testing to train the bot better every time.
In the worst stage, the call gets transferred to the customer care support with the noted logs of the conversation. A smart bot would wisely transfer to avoid more complexities.
To overcome background noise, the data is handled on form side, accepting only the values suiting the data type and capturing only the keywords trained along with their various synonyms.
A quick expert
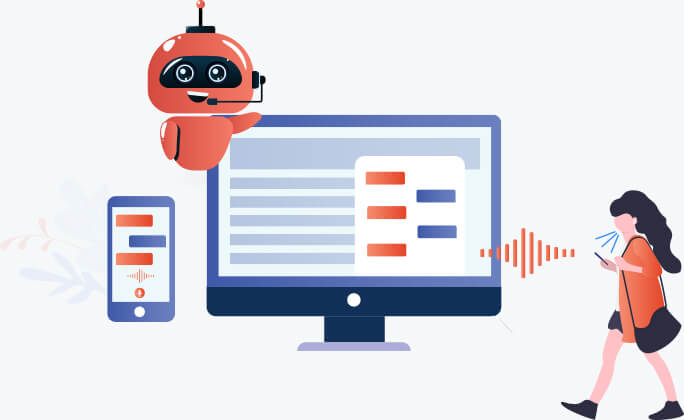
The firm has thousands of calls in one day which now can be minimalized by chatbots using the “Speech to Text” conversation along with populating the databases in the backend supporting the correct form of datatype. Using the traditional approach, of course the support team had to be available right away to solve the major error and analysis wouldn’t have been possible. With populated databases the data is now monitored along with quick analysis. The company could now handle influx of visitors and increase its sales rate abruptly by staying in the market with the latest technologies.